Downtime is one of the leading threats to software businesses. It costs organizations $5,600 per minute on average and is the culprit behind meaningful drops in customer satisfaction scores. In 2021, Meta publicly shared that a six-hour outage translated into a loss of about $65 million! For these reasons, organizations have been known to dedicate around 20-30% of their annual infrastructure software budgets towards observability, which may translate into millions of dollars for some larger organizations. Given the mission-criticality of observability tools, it’s no surprise that the observability market represents one of the most mature markets within infrastructure software. Applications and infrastructure are constantly changing and becoming more complex and distributed, pushing organizations to adopt more sophisticated observability tools to ensure the reliability of their underlying systems.
To rapidly troubleshoot system issues or respond to production incidents, many companies establish dedicated observability teams comprised of site reliability engineers, platform engineers, and other specialized roles. These teams are responsible for leveraging observability tools to meet service-level metrics around uptime and availability (e.g., SLA/SLO/SLI) and incident resolution time (e.g., MTTR). Because observability tools are only as good as the data they collect, observability teams tend to place a great deal of emphasis on extracting observability data across various data modalities — metrics, logs, and traces — from systems in a process commonly known as instrumentation.
Understanding the key components of an observability stack
With organizations frequently stitching together four or more tools to create their observability stacks, it’s worth understanding the key components and the role each component plays in helping organizations effectively monitor their underlying systems.
A generalized view of the modern observability stack is shown in the diagram below, which contains the following components:
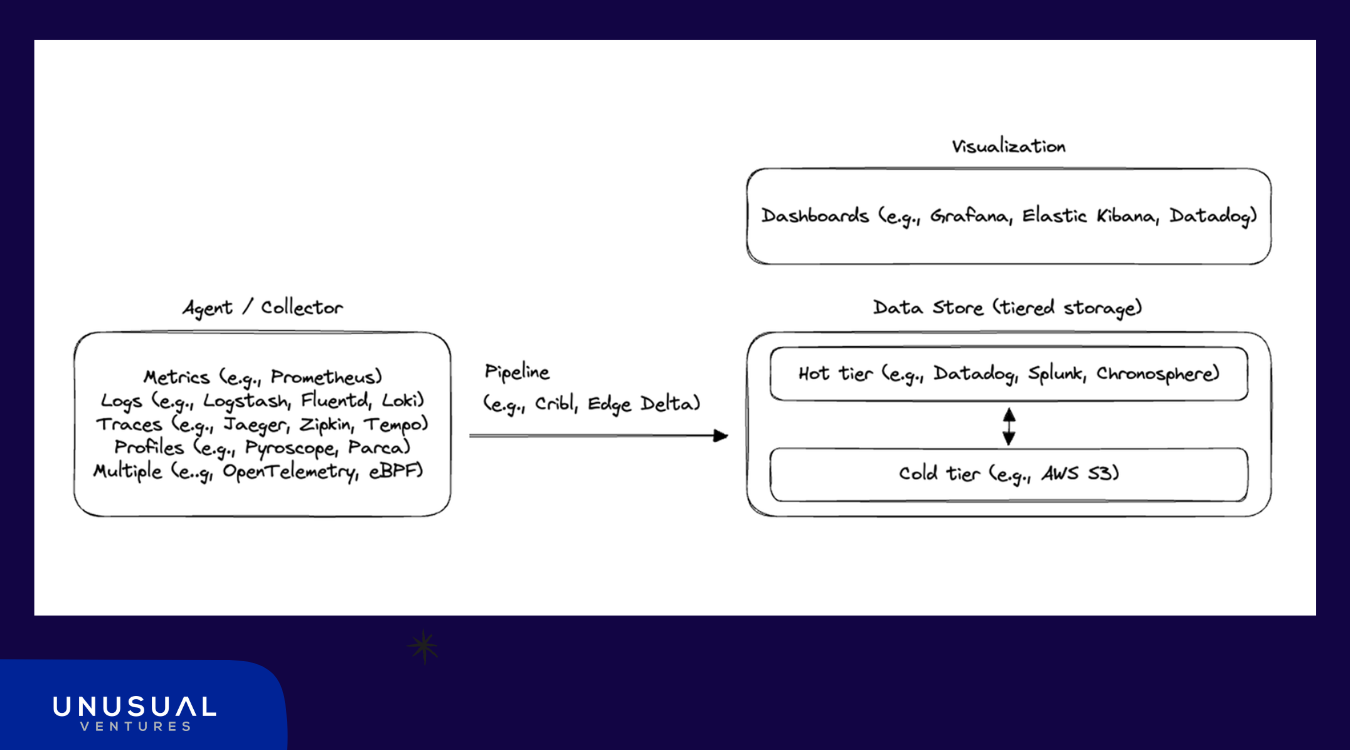
- Agent / Collector: As part of the instrumentation process, observability teams deploy specialized agents that help aggregate and collect different types of observability data from systems. Agents are typically open-source, given that they are deployed into both cloud and on-premise environments where applications and infrastructure live. Although instrumentation has historically been seen as an onerous process that often involved deploying multiple agents and importing numerous client libraries, newer projects such as OpenTelemetry and eBPF drastically simplify this process through a unified standard and toolchain.
- Pipeline: Observability pipelines transform, filter, and route observability data moving from source to destination. They are cleverly placed in front of observability data stores to pre-process data, resulting in less data storage and considerable cost savings. By leveraging observability pipeline vendors such as Cribl and Edge Delta, organizations have been able to successfully take more control over the footprint of their observability data.
- Data Store: Serving as the source of truth for observability data within organizations, data stores often consist of both databases and object storage. The data storage type is determined by the type of observability data that is being stored and its corresponding data volume. As data volumes proliferate, observability teams may choose to convert to a tiered storage architecture where less frequently accessed data can be moved from observability vendors such as Datadog and Splunk to cheaper storage options such as AWS S3 and ClickHouse.
- Visualization: Visualization encompasses the set of user interfaces by which observability teams explore and analyze observability data. To facilitate workflows, teams maintain a variety of dashboards unique to the systems and data types that are being monitored. Separately, visualization tools offer interfaces that allow engineers to quickly query the data they need for analysis. Popular visualization tools such as Grafana Dashboards provide clean out-of-the-box experiences for observability teams that are looking to make sense of their observability data.
Mapping new trends in observability
Looking at the current observability landscape may prompt the question: why are there so many observability tools? The reality is that the observability market has existed for decades and has constantly needed to evolve to solve the challenges presented by new application and infrastructure architectures.
The evolution of observability can be best illustrated by several key phases that we’ve highlighted in the diagram below:
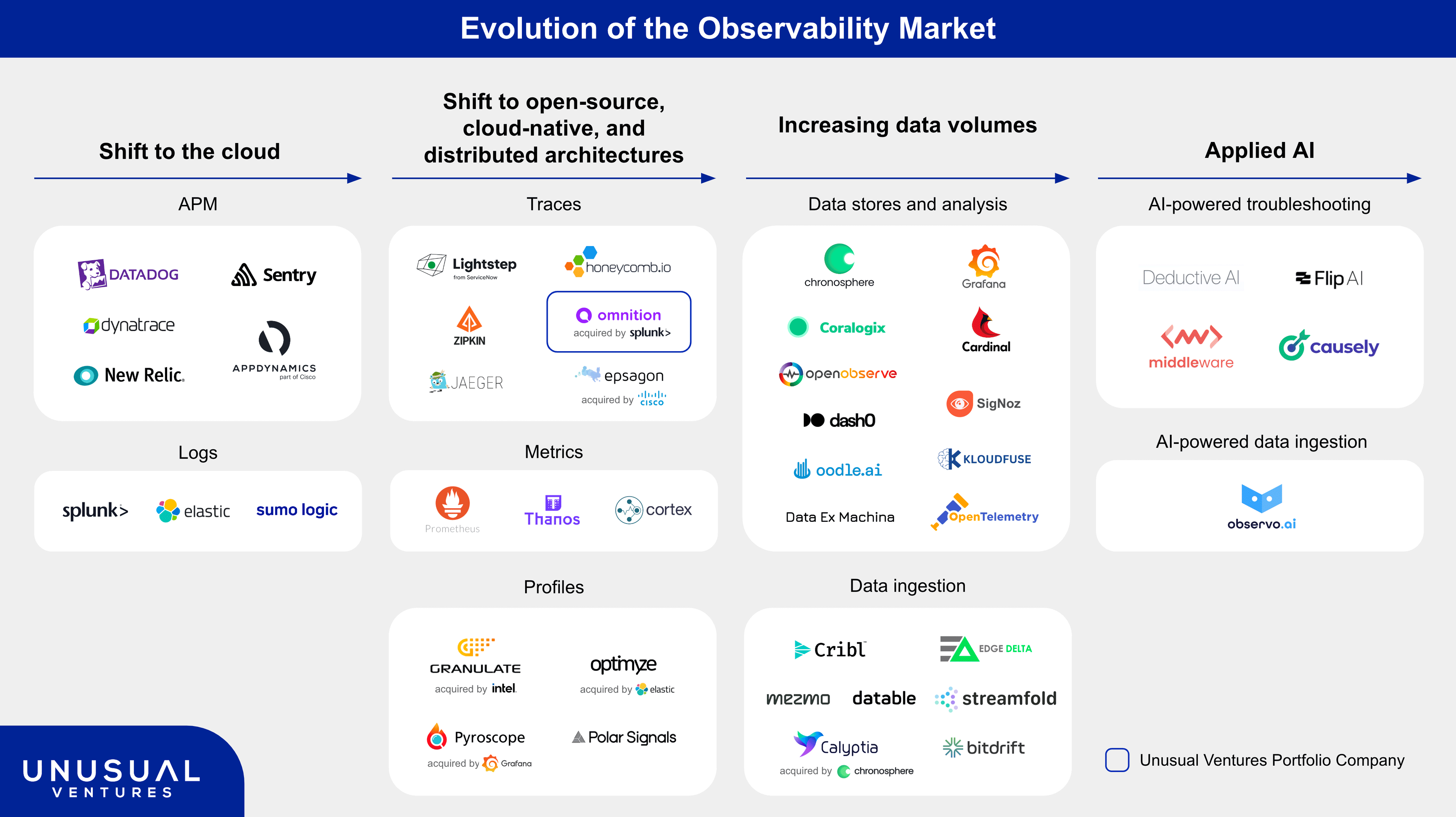
As the observability market has matured, there has been more emphasis placed on newer workflows surrounding the data store, as shown on the right side of the diagram. Companies such as Deductive AI are introducing new AI-powered tools for troubleshooting and incident response. Separately, companies such as Observo AI are allowing observability teams to perform intelligent pre-processing of observability data.
In this latest chapter of observability, here are a few trends that we’re paying close attention to:
Continued adoption of open-source and open standards
Widely adopted open-source projects such as Prometheus and rapidly growing open standards such as OpenTelemetry have ushered in a new generation of observability stacks that are modular and interoperable, as shown in case studies published by companies such as eBay. With OpenTelemetry well on the path to becoming a graduated project within the Cloud Native Computing Foundation, it’s clear that the future of observability will be open by default.
Vendor consolidation
Continued compression in observability budgets has caused organizations to carefully assess the mission-criticality of their observability tools. Observability teams are constantly being told to “do more with less” by squeezing as much value out of their existing observability tools as opposed to adding new point solutions to their observability stacks.
Serverless architectures and pricing models
Eye-catching headlines such as Coinbase’s $65 million Datadog bill have created a new status quo around serverless architectures and pay-per-use pricing models. As a result of new and existing observability vendors moving to fully embrace this new status quo, cheaper costs for querying and storing observability data have become table stakes.
AI in observability
Rapid advancements in Large Language Models (LLMs) that are trained or fine-tuned on observability data have reignited excitement around the potential for AI to accelerate workflows such as Root Cause Analysis (RCA). Although we are still far from fully autonomous RCA leveraging AI agents, new AI-enabled tools such as Flip AI are focused on making it a reality.
Our team at Unusual has a long track record in observability dating back to the early days of Unusual Co-founder Jyoti Bansal’s first company, AppDynamics. We continue to be excited about new opportunities to innovate within observability.
If you’re building in observability, we’d love to hear from you — please reach out to simon@unusual.vc.
Lorem ipsum dolor sit amet, consectetur adipiscing elit. Suspendisse varius enim in eros elementum tristique. Duis cursus, mi quis viverra ornare, eros dolor interdum nulla, ut commodo diam libero vitae erat. Aenean faucibus nibh et justo cursus id rutrum lorem imperdiet. Nunc ut sem vitae risus tristique posuere.