How startups can design bleeding-edge AI-native SaaS for enterprise
Over the past few years, millions of us around the world have interacted with AI applications emerging from tech giants like Google, Microsoft, et al. The progress and breakthroughs have been fast, frequent, and very visible, particularly in predictive technology — from Amazon (collaborative filtering) and Netflix (recommender) to TikTok’s “fyp,” companies made huge advances in statistics every day.
We saw Deepmind’s AlphaGo beat human players in 2015. However, apps that attempted to talk or act like humans still felt very gimmicky, like a novel trick that’s entertaining to mess with after a long day of work. It wasn’t easy to see exactly how we would go from Alexa playing songs in 2015 to nearly a million people using Generative AI software like Jasper to create content in 2022.
The big breakthrough in “modern AI” came in 2017 with Google’s Transformer, a novel neural net for understanding language. This has been the basis of many foundational models, particularly LLMs (large language models) that are being built across dozens of companies including OpenAI, Stability AI, and Hugging Face, etc. While interest in AI-native software seems to have exploded in the past few months, thousands of developers have been building modern AI tech and making steady breakthroughs since 2017.
The rise of these LLMs will impact everything from software to healthcare, robotics, and the way we think about the concept of human knowledge itself. It’s been an inspiring five years.
There’s a disturbance in the SaaS force
So why the sudden hype around AI-native SaaS? In the past few months of 2022, several foundational models like Stable Diffusion have been open-sourced with APIs, making generative AI infrastructure 10 to 100X more accessible. For the first time, it’s fast, cheap, and easy to build on for developers everywhere. Hundreds of new startups have sprung up or pivoted to building tools that leverage these open-source models (see CarperAI) or even closed-source ML-as-a-service platforms like OpenAI.
This has brought us to the third wave of Applied AI in SaaS: generative software. The first wave was predictive ML — software that analyzed vast amounts of data to predict an outcome or recommend a solution. For example; 6Sense processes 1-2TB of data every day for revenue ops teams. The second wave has been in cognitive tools that not only predict an outcome but then automatically act on our behalf. Examples of these span from cybersecurity software to the operating systems for self-driving cars. The third wave is that of generative software — scaling unique human-like work output across modalities, including text, image, voice, code, music, 3D models, etc. More than a million developers have already used Github Copilot to preview AI-generated code.
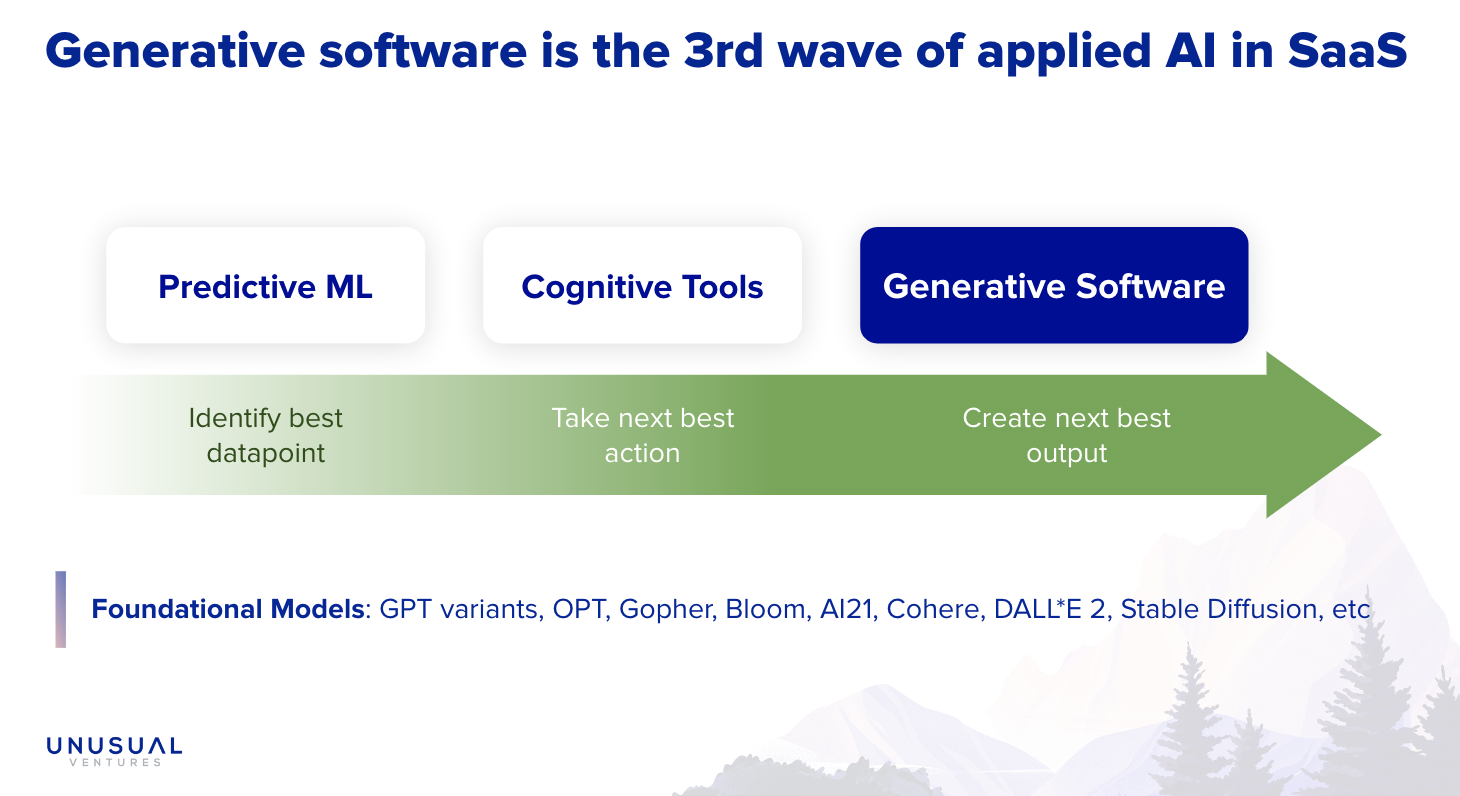
Will AI put us all out of work? No, not yet.
Since the industrial revolution, human expertise and creativity have always found a way to create value in our world. Generative AI will expand human potential. For example, for the first time, I could perhaps produce good dubstep music using Magenta. It will be unique and not technically plagiarized. It will also not be original or good enough for me to turn pro. However, it would be a new act of creativity that was previously out of my reach. In fact, Fabian Stelzer did something just like this with SALT, an entire '70s-style sci-fi movie generated using AI by someone who had never produced a film before.
What else could you apply this framework to? What could it do for human productivity and aspirations?
Is 2023 the year AI-native SaaS crosses the chasm?
While generative AI models already feel extraordinarily magical, they are only the starting point for enterprise SaaS. The core reason for this is that “unique” output is not the same as effective work.
And therein lies the opportunity for developers building AI-native software based on these powerful open-source models. For generative AI to cross the chasm to enterprise software, founders will need to address four core pillars that will become their technical moat:
- Team workflows: how does the software work seamlessly within your users’ context?
- Governance and ethics: how does it offer control, build trust, avoid plagiarism and bias?
- Active learning: how does it let users proactively train models to fit custom needs?
- Multimodal analytics: how do you quantify the quality and effectiveness of each piece of work?
Building generational AI SaaS companies that address these four needs requires a deep understanding of your customers’ context and problems. As founders building on top of open-source foundation models, this, along with speed of market execution, becomes your IP and competitive advantage.
The 4 pillars of enterprise-grade generative AI software
1. Team workflows
a. Integrated UX: let users leverage your AI tool within their primary, locked-in system of work. Note: this is not a need for new consumer software, it’s quite the opposite — you want users to switch to a new, AI-native workflow.
b. Collaboration: make sure an individual user can get started on their own before they bring team members in with distinct roles.
2. Governance and ethics
a. Control vs. trust: prevent users from over-trusting results by offering them enough control and transparency that they have the right expectations from the tool.
b. Lineage: help users avoid plagiarism and putting their companies/employers at risk of litigation when using AI tools.
c. Bias: offer explicit metadata for possible bias in training data where needed.
3. Active learning and tuning
a. Fine-tuning: help users leverage models for adjacent tasks.
b. Updating: create ways to let users proactively add new training data to existing models.
4. Multimodal analytics
a. Quality: quantify the attributes of the end result that matter — how unique is it? How funny? How optimistic?
b. Effectiveness: create a clear feedback loop to track the effectiveness of the output.
Investing in AI-native SaaS
At Unusual, we have made several early-stage investments in AI-native SaaS companies in the past two years, including seed investments in Orum (automated sales calls), RelicX (generates software tests), Enterpret (synthesizes customer feedback), and Mem Labs (self-organizing workspace). We know what it takes to find product-market fit for a new, emerging technology and prefer to lead day 0 investments with $2-$5M cheques. If you are looking for a great team of operator-VCs to help you dominate enterprise software with generative AI technology, we would love to work with you!
Lorem ipsum dolor sit amet, consectetur adipiscing elit. Suspendisse varius enim in eros elementum tristique. Duis cursus, mi quis viverra ornare, eros dolor interdum nulla, ut commodo diam libero vitae erat. Aenean faucibus nibh et justo cursus id rutrum lorem imperdiet. Nunc ut sem vitae risus tristique posuere.